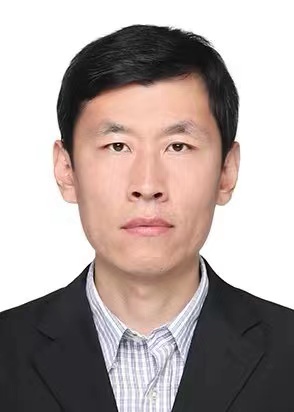
常海滨,男,辽宁清原人,1983年12月生。本科毕业于吉林大学数学学院,博士毕业于北京大学工学院,之后在北京大学从事了博士后和研究岗位工作,于2022年6月入职12bet(北京)12博BET官方网站。主要研究方向为渗流数值模拟、开发优化、数据同化和机器学习。代表性成果:(1)提出了复杂储层介质(如岩相、裂缝、渠道)的反演算法;(2)提出了用于强非线性问题(如流固耦合问题)的迭代数据同化算法;(3)提出了数据驱动的物理规律挖掘方法;(4)提出了融合领域知识的深度学习方法。在Journal of Computational Physics、Computer Methods in Applied Mechanics and Engineering、Journal of Geophysical Research: Solid Earth、SPE Journal和Journal of Hydrology等国际知名杂志发表SCI论文20余篇。担任10余国际知名期刊审稿人。获授权国家发明专利1项。主持/参与博士后基金、国家自然科学基金、国家科技重大专项子课题和横向课题等项目10余项。
Short Biosketch of Dr. Haibin Chang
Haibin Chang was born in 1983, Liaoning, China. He received bachelor’s degree in mechanics from Jilin University, China, and received PhD degree in energy and resources engineering from Peking University, China. After that he sequentially worked as post doctor and research scientist in Peking University. He joined China University of Mining and Technology (Beijing) in June 2022. His research interests include subsurface flow simulation, production optimization, data assimilation, and machine learning.Hepublishedover 20journal papers, which are published in high quality journals, such as《Journal of Computational Physics》,《Computer Methods in Applied Mechanics and Engineering》,《Journal of Geophysical Research: Solid Earth》,《SPE Journal》and《Journal of Hydrology》.His 10 representative papers are listed below.
代表性论文:
[1] Wang, N., Chang, H. *, Zhang, D. *, Efficient uncertainty quantification for dynamic subsurface flow with surrogate by Theory-guided Neural Network. Computer Methods in Applied Mechanics and Engineering. 2021, 373, 113492.
[2] Wang, N., Chang, H. *, Zhang, D. *, Deep-Learning-Based Inverse Modeling Approaches: A Subsurface Flow Example. Journal of Geophysical Research: Solid Earth. 2021, 126(2), e2020JB020549.
[3]Xu, H., Chang, H. *, Zhang, D.*, DLGA-PDE: Discovery of PDEs with incomplete candidate library via combination of deep learning and genetic algorithm. Journal of Computational Physics. 2020, 584, 124700.
[4]Chang, H., Zhang, D., Machine learning subsurface flow equations from data. Computational Geosciences. 2019, 23(5), 895-910.
[5]Chang, H., Zhang, D., Identification of physical processes via combined data-driven and data-assimilation methods. Journal of Computational Physics. 2019, 393, 337-350.
[6]Chang, H., Zhang, D., History matching of stimulated reservoir volume of shale gas reservoirs using an iterative ensemble smoother. SPE Journal. 2018, 23(2), 346 - 366.
[7]Chang, H., Liao, Q., Zhang, D., Surrogate model based iterative ensemble smoother for subsurface flow data assimilation. Advances in Water Resources. 2017, 100, 96-108.
[8]Chang, H., Liao, Q., Zhang, D., Benchmark problems for subsurface flow uncertainty quantification. Journal of Hydrology. 2015, 531, 168-186.
[9]Chang, H., Zhang, D., Lu, Z., History matching of facies distribution with the EnKF and level set parameterization. Journal of Computational Physics. 2010, 229, 8011-8030.
[10]Chang, H., Chen, Y., Zhang, D., Data Assimilation of Coupled Fluid Flow and Geomechanics Using the Ensemble Kalman Filter. SPE Journal. 2010, 15(2), 382-394.
Contact: Haibin Chang
School of Energy and Mining Engineering
China University of Mining and Technology (Beijing)
Ding 11 Xueyuan Road, Beijing 100083, P. R. China
Email: hb-chang@163.com